Why the Rise of Donald Trump Should Make us Doubt the Hype About Artificial Intelligence
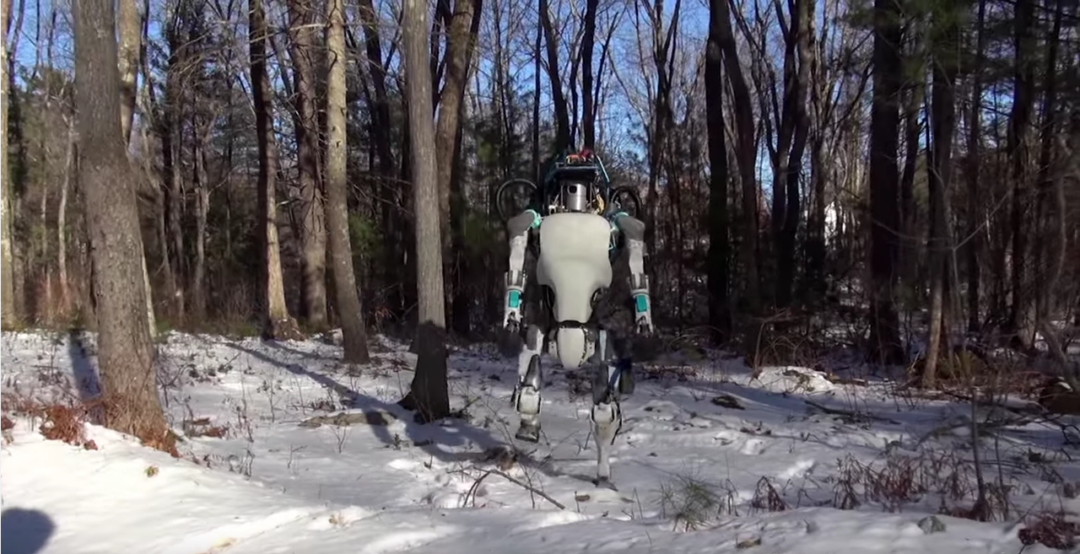
By March 28, 2016
\As the Primary season has progressed, there’s been no end of political pundits backpedaling and mea-culpa-ing over their previous inability to predict the rise of Donald Trump to become the frontrunner in the GOP. From Charles Krauthammer admitting that it was wrong to laugh at The Donald to innumerable others, both liberal and conservative, wishing they’d take Trump seriously, it seems like just about everyone in the Predictive Class will be dining on roast crow this Easter.
But why did they get things so wrong? Was it because they assumed that he’d “crash and burn” like John Podhoretz? Was it because they assumed that he couldn’t win because Republican voters hated him, as implied by Patrick Murray of Monmouth University when releasing early poll results in June of 2015? Or was it just because that everyone assumed that Jeb Bush would win the nomination?
Yes. And no. The truth is, nobody really knows why nearly every professional political predictor got things wrong. But, as we near the end of March, it looks like Trump is going to defy conventional wisdom and – all threats of 3rd parties or brokered conventions aside—win enough delegates to clinch the nomination as the Republican Party’s next presidential candidate.
So how could so many smart people have been wrong about Trump? After all, these aren’t barroom loudmouths spouting off at the local watering hole…these are seasoned political operatives with, in most cases, decades of experience making a living predicting the winners and calling the losers before the rest of us. Yet even with all their experience, even with all the information available to them online, even with access to the vast 24/7 poll that we like to call “social media,” most of them totally missed the mark.
Of course, it’s always easy to be a Monday morning quarterback about such things, but if we look back over the past year, it’s possible to pluck out a few patterns. First was their insularity: for the most part the well-known celebrities of punditry tend to hang out in somewhat more rarefied air than the rest of us. As New York Times columnist David Brooks admitted on NPR recently, “I was wrong. And I think it’s because I wasn’t socially intermingling with the sort of people who are Trump supporters…I’ve got to spend more time with different sorts of people.”
Another reason might just be the “filter bubble” effect where what we see online becomes narrower and narrower the more we use the ‘net as a result of algorithms at work in search engines, social media sites, and even consumer e-commerce sites. While they’re ostensibly well-intentioned attempts at feeding each of us the information that’s most relevant to us, the end result is that we don’t end up seeing anything that challenges our existing worldviews. While there’s lots of information out there, much of it is imperfect.
But the real reason might be a lot simpler: people just aren’t that easy to predict. History is rife with examples of “experts” missing the boat. From the spectacularly nerdtastic rise and (literal) fall of the much-vaunted Segway, to the innumerable failures of technology to magically transform education (remember the whole “One Laptop Per Child” hype?), to the unforeseen consequences of US intervention in WW 1 and the horror that followed Germany’s humiliation mandated by the Treaty of Versailles at the end of that war and so many other examples both tragic and mundane, it seems that people are pretty lousy at predicting the future behavior of other people.
If you really think about it, our collective track record as predictors of human behavior shouldn’t be such a surprise. After all, as anyone with children knows, it’s pretty hard to predict what your own flesh and blood will do when presented with a novel situation, much less make predictions about the behavior of millions you’ve never met. Sure, there are plenty of situations where human beings individually might act in a predictable manner – after all, grifters, magicians, and professional “psychics” make their living off of the predictability of individuals in controlled situations—but when it comes to being able to predict what humans are going to do in an accurate and repeatable manner we might as well be flipping coins…and, as recent research shows, coin flips might do a better job than the “experts.”
So if we’re so bad at predicting behavior, why is it that so many experts seem to think that we’re going to be able to program computers to become as intelligent – or more intelligent—than us?
It’s been pretty hard lately to avoid the hype about the breakthroughs in artificial intelligence that are supposedly right around the corner. From the techno-utopianism of Ray Kurzweil’s “singularity” to the dire warnings about the dangers of unchecked AI coming from science and technology luminaries like Elon Musk, Stephen Hawking, and Bill Gates to growing fears that AI will automate nearly everyone out of a job leaving nothing but “gods and the rest of us,” artificial intelligence has leaped out academic obscurity and into the public consciousness.
It’s not hard to see why. Self-driving cars are predicted to be a fact of life within the next 4 years. Personal digital assistants that we can carry in our pockets and activate with our voice have become nearly ubiquitous. Many of us have basically turned our investing over to automated trading systems that have become so sophisticated that we may not even understand how they work anymore. Robots build our cars and automated voice-activated telephone systems handle our complaints when we have problems. Soon, automated flying robots might be delivering our packages. It seems pretty obvious that the era of AI is here, or at least hovering nearby.
But are these really examples of artificial intelligence? For decades, computer scientists have been divided over the difference between “hard AI” and “soft AI,” or, as Middlesex University Professor of Artificial Intelligence Chris Huyck puts it, “doing it the way people do it” (hard AI) and “doing anything that works” (soft AI). While both approaches have their adherents, it’s hard AI that’s the Holy Grail (and the potential doom for humanity). When we have computers that do things the way we do, then we’ll have achieved “true” AI.
There’s just one problem. It may be impossible.
Right now, all the examples of what we call “artificial intelligence” are examples of “soft AI.” Systems that can drive cars, recognize faces or voices, fly on their own, make investment decisions, and all the other things that seem “intelligent” do what they do by way of algorithms and networks that “learn” in a highly specialized way. They may make actual decisions, but those decisions – or at least the rules that make them happen—have already been pre-programmed by human beings. There are some systems that use generative algorithms to “evolve” solutions to specific problems like walking, but they do so through trial and error, keeping what works and discarding what doesn’t until they’ve “figured out” how to solve the problem they were tasked with solving.
These systems may seem smart to the point that they start crossing over into the “uncanny valley” of being a little too human-like but unlike us, they’re only smart about one thing. A self-driving car might be orders of magnitude better at navigating roadways than most humans, but ask it to do some high frequency trading for you and it’ll just sit there. Likewise, a drone might be able to zip through the forest by itself avoiding obstacles at 30 miles per hour, but ask that drone to diagnose simple medical problems or offer a recommendation about how to optimize your next ad buy and you’ll quickly find out that you might as well ask a bird for help. “AI’s” as we know them today are basically one trick…errr…whatever this is.
One fact that anyone who has ever programmed a computer will tell you is that computers are stupid. They only do what they’re told. They can be programmed to simulate behavior that appears “intelligent” or even loaded up with algorithms and neural networks that allow them to operate autonomously within a limited realm (driving, flying, buying and selling stocks), but present them with a novel situation outside of the domain that they’ve been programmed for and all of a sudden they don’t seem so smart.
To date, most researchers pursuing the dream of hard AI have come from the rationalist/mechanistic viewpoint that asserts that humans are just a collection of parts and that our brains are just like computers, only a lot faster and more capable of learning. This view, popularized by futurist and inventor Ray Kurzweil, basically states that artificial intelligence will happen when computers are capable of the same number of interconnections and the same level of pattern recognition humans have (approximately 300 million pattern recognizers, if you must ask). We’re not there now, but due to Moore’s Law and the pace of technological change, the time when computers achieve the same processing power as the human brain is just around the corner…maybe even the next 5-10 years. After that point computers will be able to design themselves and all bets are off about what happens then…it’s impossible to see into the future past this “singularity” point.
Or maybe not. Recent research has revealed that the average human brain can store more information than the entire World Wide Web. Attempts to simulate the human brain through computer hardware are facing increasing criticism for their reliance on outdated models that stretch the equivalences between brains and computers. And even though it may be possible to have a conversation of sorts with Siri on your iPhone, she’s got a long way to go before she could pass the Turing Test.
But the chasm that has to be crossed before achieving hard AI might be a heck of a lot deeper than issues around simulating or modeling the human brain as a pathway to intelligence. In fact, as philosopher David Searle asserts, while we may someday have computers powerful enough and programs sophisticated enough to simulate human intelligence, they still won’t actually understand what they’re doing in the same way we as humans are conscious of ourselves. We may be able to write enough rules, he asserts, to create the “correct” outputs from the inputs the “intelligent” computer receives, but following rules isn’t real “intelligence.”
In many ways, the failure of so many all-too-human “experts” to predict Donald Trump’s popularity illustrates why achieving hard AI may be impossible. If a set of human beings, trained in predicting the behavior of other human beings in a specific domain—politics—have failed, how could we ever program a computer to do better? After all, wouldn’t that computer have to be programmed with knowledge and rules from experts in order to do its predicting? And if these experts don’t even really know how they do what they do (or, maybe, how to really do it), then how could a computer do better if it only had access to the same information that the experts had?
Of course, asking questions that assume that rules, experience, knowledge, and access to information are what makes up “intelligence” falls into the same trap that those who think that “all” we have to do to achieve intelligence is have computers that operate on the same basic hardware patterns as the human brain. Once we can basically create a brain in a jar, that brain will be intelligent, right?
Regardless of what movies may (or may not) have taught us, we’re not brains in jars. Sure, the brain might run the show, but it exists in the context of the body and that context may be the key to what “intelligence” in the human sense really is.
So what defines “intelligence?” What separates humans from the “non-sentient” creatures we share the planet with? While these are philosophical topics that have consumed centuries of debate and thought, it’s pretty safe to assert that one core element that separates humans from other animals is our ability to communicate with one another. Yes, there’s evidence that animals such as apes, whales, dolphins, and even crows may have limited communication with others in their species, but even granted that, it’d be ridiculous to not admit that humans are able to communicate at a vastly higher level. In fact, the only way individuals know anyone else is conscious in the same what that we perceive ourselves as being conscious, is through communication.
But communication isn’t just sounds (speech), graphical marks (writing) or even representation and expression (the arts). Communication in its fullest sense – the sense that we encounter when communicating with someone in person—is mostly non-verbal. Body language, physical contact, facial expressions, and even smells all combine together to communicate in a way that speech or writing alone (or even video) can’t. As anyone who’s got into trouble by attempting to use sarcasm in email knows, it’s pretty easy to miscommunicate when you’re limited to one modality. That’s why we have emojis.
Emojis work because they add additional visual information to our communication that helps the person we’re trying to communicate with transcend the base textual information in the messages we send. In person (or even over good two-way video), eye contact (or lack thereof) can do more to communicate than many minutes of talking (or many written words) can. A touch on the arm communicates something that can’t be replicated through any other method of communication because of the feelings it generates in both the person doing the touching and the person being touched.
Computers might be one of humanity’s greatest technological achievements but they lack one thing that we as humans have: a body. And while computers may be made to read human facial expressions, parse out spoke words, or even be able to detect human moods based on affective phenomena, being able to detect isn’t the same as being able to understand. Fully understanding human communication in its fullest sense requires a body because it’s our body that allows us to have the kind of empathy necessary to understand non-verbal communication. There are forms of communication that can’t be put into words but can be transmitted, received, and understood because we as humans are able to count on our shared experience as humans. When we see pain or joy or puzzlement on the face of another person we understand what they’re communicating to the world not because we can detect specific facial movements indicating an emotion — although that’s certainly part of understanding– but because we ourselves have felt similar emotions. There may be no way of actually knowing what someone else’s subjective experience “actually” is, but the empathy of shared experience helps us to understand in a way that transcends the information being transmitted. There’s a big difference between reading that it may hurt to bump your toe into bedpost and actually stubbing your toe, after all.
David Brooks said that he missed the boat with Trump because he “wasn’t socially intermingling with the sort of people who are Trump supporters.” And if he couldn’t truly understand Trump supporters because he wasn’t hanging out with them, how much worse would a computer without a body – or, possibly worse, a non-human body—do when it comes to intelligently predicting human behavior? Until we can know that, we’re a long way off from hard AI.
Sean leads our Discover360 engagements, gathering data and research to develop the insights necessary for crafting effective strategies for our clients. He has a perfectly varied background for our higher education and nonprofit partners: He’s served as everything from a dean to an adjunct professor to the co-director of a high school cybersecurity summer camp to the leader of a university 3D printing lab. Sean also has an uncanny talent for creating the perfect meme faster than you can search for one.